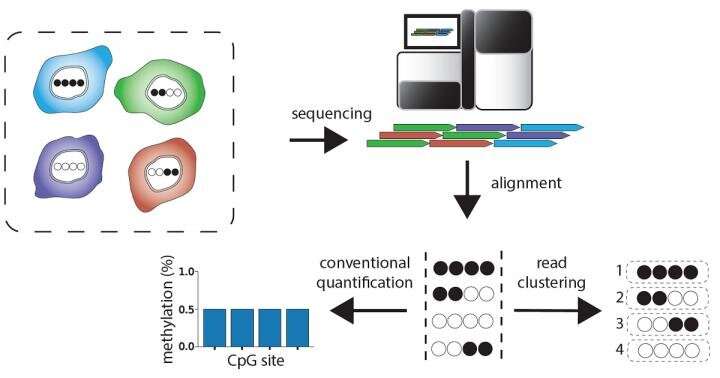
There are about 75 different types of cells in the human brain. What makes them all different? Researchers at Baylor College of Medicine have developed a new set of computational tools to help answer this question. Although different cell types from the same organism carry the same DNA, they look and function differently because a different set of genes is active or inactive in each. Cells switch genes on or off by using epigenetic mechanisms, such as DNA methylation, which involves tagging genes with methyl chemical groups.
To better understand how epigenetic regulation works, researchers study DNA methylation signals in whole genome datasets. These datasets contain the sequences of the building blocks that make up the DNA in a cell population. However, when the tissue being studied, like the brain, is made up of many different cell types, existing analytical approaches can not distinguish methylation signals arising from those different cell types.
Now, a new set of computational methods developed at Baylor allows researchers to identify cell-type specific methylation patterns—molecular barcodes—in complex cell mixtures. These new computational tools, published in the journal Genome Biology and available for free download, can be applied to existing whole-genome methylation datasets from any species. This opens exciting new possibilities to improve our understanding of how DNA methylation regulates cellular function.
Identifying cell type-specific molecular barcodes
“The current gold-standard approach to study DNA methylation is whole genome bisulfite sequencing (WGBS), a next-generation sequencing technology that determines DNA methylation of each cytosine, one of the DNA building blocks, in the entire genome,” said co-corresponding author Dr. Cristian Coarfa, associate professor of molecular and cellular biology and part of the Center for Precision Environmental Health at Baylor.
WGBS studies typically report the average methylation level at each cytosine. In tissues made up of multiple cell types, however, this average reflects a mashup of the methylation level of each cell type in the mixture, obscuring cell-type specific differences.
“The key insight that motivated the current study is that the DNA sequence ‘reads’ in WGBS data are direct descendants of DNA molecules originating from different cells of the tissue. We postulated that the methylation ‘patterns’ we detect on tissue sequencing reads contain information about what cell types the reads originated from,” said co-corresponding author Dr. Robert A. Waterland, professor of pediatrics—nutrition at the USDA/ARS Children’s Nutrition Research Center at Baylor and Texas Children’s Hospital. “To test this we developed software that identifies these cell type-specific methylation patterns within bulk WGBS data. This software is called Cluster-Based analysis of CpG methylation (CluBCpG).”
As one validation, the researchers used CluBCpG to analyze WGBS datasets from two types of human immune cells, B cells and monocytes. They were able to identify over 100,000 unique molecular barcodes within each cell type. Then, they applied their method to mixtures of reads from another WGBS dataset from these two cell types, from entirely different people.
“Just by counting occurrences of these molecular barcodes in the novel datasets, CluBCpG allowed us to precisely determine the percentage of B cells and monocytes in each mixture,” said Dr. C. Anthony Scott, former postdoctoral researcher in the Waterland lab and co-first author on the paper. “We also showed that these cell-type specific signals are associated with cellular functions in different types of human and mouse brain cells and blood cells, and that they can even predict which genes are expressed.”
In the last 10 years, scientists generated thousands of WGBS data sets costing millions of dollars, yet were unable to appreciate much of the information available in the data. “It’s a bit like wearing noise-cancelling headphones to the symphony,” said Waterland, also a professor of molecular and human genetics at Baylor. “Now, for the first time, researchers can ‘tune in’ to the full richness and complexity of WGBS data.”
Boosting the information content of existing datasets
The CluBCpG software works together with a second development, a sophisticated machine-learning software package called Precise Read-Level Imputation of Methylation (PReLIM). This software ‘fills in’ missing information on sequencing reads that cover some of the sites in a region, increasing the information content of existing WGBS datasets by 50 to 100 percent.
“PReLIM learns from the hundreds of millions of reads in each WGBS dataset to predict the methylation state at missing sites on individual sequence reads,” said Jack D. Duryea, former student in the Waterland lab and co-first author on the paper. “We showed that PReLIM’s predictions are correct 95 percent of the time.”
Since WGBS datasets cost thousands of dollars to generate, getting 50 to 100 percent more data—at no extra charge—is a big deal.
The researchers anticipate these new computational developments will be applied to study methylation differences in normal cells as well as in disease.
“For instance, these methods will provide better resolution in studies aiming to identify methylation differences between a healthy brain and one with a disease. We might be able to determine, for example, that epigenetic changes linked to a disease occur only in one specific type of brain cell, which would be a major step toward understanding a disease,” Waterland said.
A treasure map to understanding the epigenetic causes of disease
C. Anthony Scott et al, Identification of cell type-specific methylation signals in bulk whole genome bisulfite sequencing data, Genome Biology (2020). DOI: 10.1186/s13059-020-02065-5
Baylor College of Medicine
Citation:
Novel software reveals molecular barcodes that distinguish different cell types (2020, July 1)
retrieved 1 July 2020
2020-07-software-reveals-molecular-barcodes-distinguish.html
This document is subject to copyright. Apart from any fair dealing for the purpose of private study or research, no
part may be reproduced without the written permission. The content is provided for information purposes only.